Does your company produce original and captivating content? Do you work in Publishing, Advertising, or Marketing? Do you think you know exactly what your followers expect from your content? Are your strategies effective enough to encourage your readers to press the call-to-action button?
Ok. If you could answer only half of the questions, it is high time to turn to advisors. And here we are to show you the way to them.
Let us tell you that there is nothing more important for the news media than keeping their readers and subscribers engaged. And to do so, if you work in the media industry, it is mandatory for your team to pay attention to what your users generally look up to and what drives them to make that choice. Once you get familiar with their behavior, you will never again call the results of your content into question.
We are positive that high-quality software is the answer to all your questions. Thanks to Machine Learning, nowadays, it is possible to anticipate your users’ behavior and preferences. In other words, even before investing time and effort in publishing content and waiting for your audience’s reaction, you must already be certain about the success of your campaigns in advance. Were you to survey each reader, you would not obtain such genuine data as you do when you collect it from a software tool that gets that reliable information from authentic user journey maps.
Let’s stop beating around the bush because, at this moment, you must be wondering how to get that data, set realistic expectations, and maximize your users’ satisfaction. Darwoft, one of the top software development companies in Portland, can make it possible through the use of Machine Learning models. First and foremost, let’s define Machine Learning.
What is Machine Learning?
When we talk about Machine Learning, we refer to one of the branches of Artificial Intelligence and Data Science. This is not an entirely new term. Actually, it dates back to the 1950s.
Artificial intelligence systems are trained to perform really complex tasks by imitating human behavior. As a result, it can be said that it is time- and effort- saving, especially when there are tasks that go beyond human capabilities. The algorithms applied to the machine learning model are able to save a large amount of data that will be used by the same algorithms in the near future. If the model needs to solve a new problem, it will find the proper answer by following previously learned patterns. To put it in another way, the machine processes the information received and learns from that to predict the user behavior.
This was exactly one of the most challenging tasks our team had to do since they were responsible for teaching a Machine Learning model how to read the data that was obtained from a news portal and, consequently, understand and categorize that information accurately.
Continue reading if you would like to know more about this project. 👇
Project Scope
The mission was to design, create, and deliver a detailed user behavior analysis and a prediction platform that could ingest information about the users’ behavior from a script in the client’s news portal.
On the one hand, taking in the set of actions and patterns the readers show when they check the latest news allows the news portal to get reliable data about the number of common registrations, subscriptions, and unsubscriptions. On the other hand, based on the readers’ subscription status and the proper analysis of their interaction with the product and the tendencies in real-time, Darwoft’s team could segment followers, grouping them accordingly on a complete set of dashboards and widgets.
Besides, this effort to gather the existing data, analyze it, and segment the digital community of readers represents a long-term benefit for our clients since they will be able to use this platform quantitatively. In other words, they will be ready to make comparisons between months and analyze the results because of the historical information they have collected about their users, who might have maintained, upgraded, or canceled their subscription. Once our experts in software services could track this type of information, they could create models that serve to find patterns, predict the future behavior of subscribers, and categorize every user based on their loyalty and risk of unsubscription.
Challenge: Historical and Real-Time Analysis
The team had to achieve two milestones. Initially, the team needed to work on the product definition to have a minimum viable product in six months. Then, the team had to meet deadlines and show progress.
As Darwoft works with Agile Methodologies, in which flexibility and iteration are two key elements, we prioritized showing our clients every step of the work process, so they always had the chance to see the work in progress and make changes when necessary.
These milestones, set at the very beginning, defined the path of the project and the product roadmap. We needed to be wide-awake and think through our client's main challenge, which was to find an easy and understandable way to manage real-time and historical data while maintaining great performance.
So, what did Darwoft do?
Darwoft’s Full-Scale Approach
Darwoft created a platform to analyze and predict the subscriber’s behavior toward digital news media based on subscription models. In order to do so, Darwoft ran an MVP Development process.
- The beginning of the project is to share a common biz vision within the team. A kickoff was held on the first day of the project to share the common business vision of the product and the objective: Predict the future behavior of the reader in order to increase the number of subscriptions and reduce the risk of unsubscription while maximizing the loyalty of users.
- During the first month, when the Product Discovery process started, the focus was mainly on both UX design and architecture. Analyzing possible competitors and similar apps encouraged a discussion to understand the differential value of the application while helping to clarify the concept. These meetings were fundamental for Darwoft’s senior architects since they had to create an Infrastructure Pipeline, a Technical Roadmap, and a Functionalities Map to prioritize customer- and client-facing functions based on end-customer uses.
- After the flow of the application experience was fully defined, Darwoft assigned a team that included the UX designer, as well as a project manager and a senior technical leader, to finalize the architecture design and underpinning platform. The team spent three months working on development, which ended up in the first version of the product that met the expectations of the first milestone. Once all the data about the user behavior was collected, the first machine learning models were created and tested.
- After the first milestone was reached, Darwoft focused on the widgets and dashboards that were to present the data to the different users. The Darwoft team worked following the definition made in the High fidelity prototype. After that, a new version of the product was released, with the functionality for all the user personas defined.
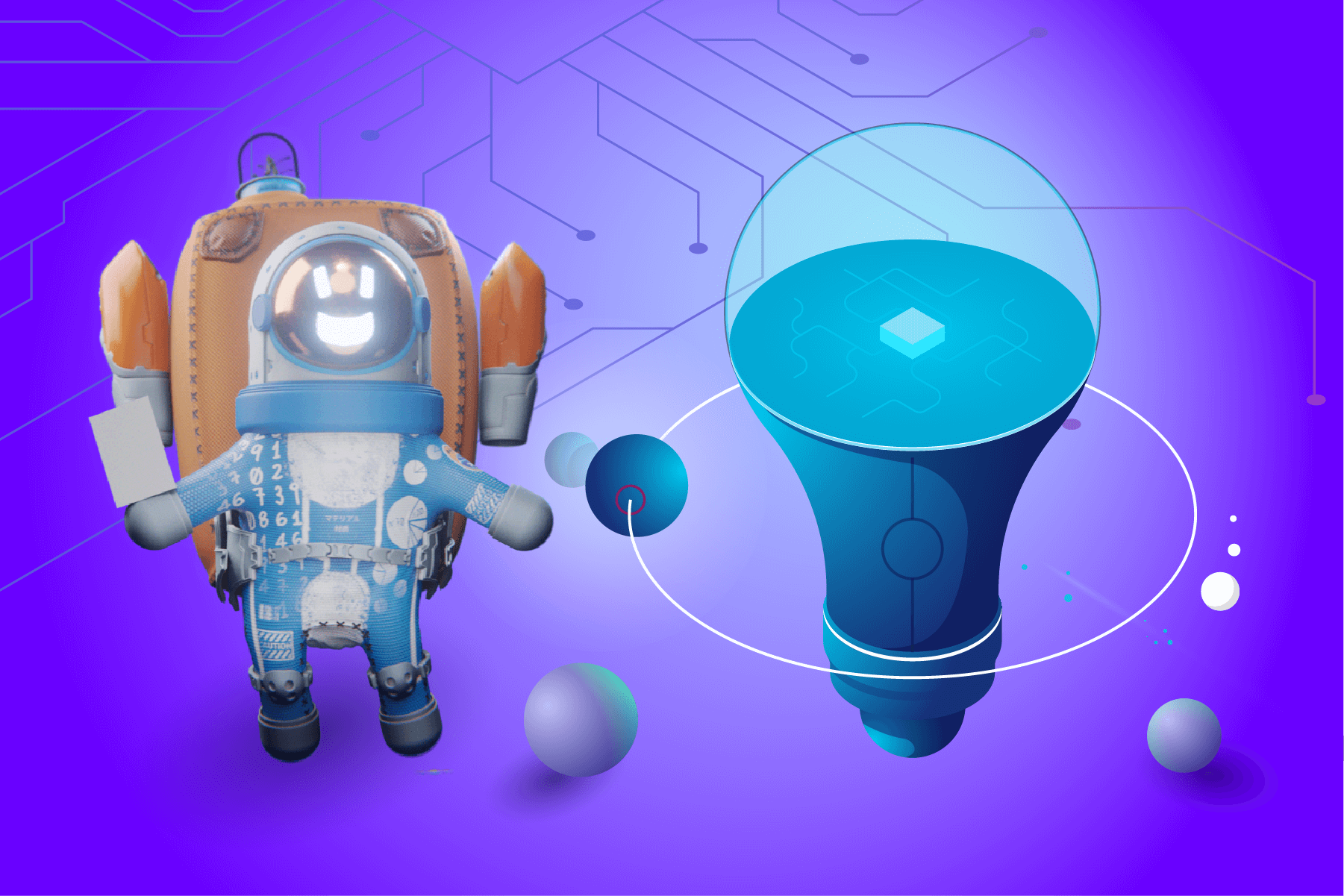
Solutions Presented by Darwoft Team
After the discovery process, the first goal was to collect all the possible data about the readers and their behavior. We needed to be able to answer the following questions:
- What sections do they usually read?
- What topics are they keen on?
- What type of articles do they like the most?
- Do they prefer reading an article or watching a video?
- What time do they read the news?
- What device do they use to read them or sign up?
Bearing this prompt in mind, Darwoft developed a JavaScript plugin capable of tracking the news portal’s user behavior and storing that information in a set of databases optimized by Analytics and Machine Learning. Besides, Darwoft created integrations between the new platform and the client’s management system, importing information about subscribers, subscription plans, notes, topics, and all the information regarding the subscriber's management.
The following month Darwoft developed a set of dashboards to present the analytics and information to the editors and marketing users, according to the designs defined during the Product Discovery phase. At that time, all the information about the users’ behavior was being collected, creating a database that was essential for machine learning models.
After some time gathering data, Darwoft created a machine learning model that classifies readers and subscribers into different groups according to their characteristics and interactions and provides predictions of future behavior after having studied their activity, loyalty, and risk of unsubscription.
Owning this information helped create a more ambitious publication schedule tailored for each group of readers and more exclusive fidelity programs aimed at increasing the subscription rates, and, at the same time, reducing the churn.
With the models running and processing information every day, Darwoft was ready to present the results in a set of dashboards for marketing users and editors.
Now, the marketing and editorial teams can understand the behavior of their audience, what type of content is the most suitable for them, and how to interact with them.
Would you like to know how your readers interact with your content?
Contact us!